144. Pytorch
基本概念
简介
模型训练5要素
- 数据:包括数据读取,数据清洗,进行数据划分和数据预处理,比如读取图片如何预处理及数据增强。
- 模型:包括构建模型模块,组织复杂网络,初始化网络参数,定义网络层。
- 损失函数:包括创建损失函数,设置损失函数超参数,根据不同任务选择合适的损失函数。
- 优化器:包括根据梯度使用某种优化器更新参数,管理模型参数,管理多个参数组实现不同学习率,调整学习率。
- 迭代训练:组织上面 4 个模块进行反复训练。包括观察训练效果,绘制 Loss/Accuracy 曲线,用 TensorBoard 进行可视化分析
张量
概念
张量
官方定义
A tensor is the primary data structure used by neural networks.
官方定义2
A PyTorch Tensor is conceptually identical to a numpy array: a Tensor is an n-dimensional array, and PyTorch provides many functions for operating on these Tensors.
通俗理解
多维数组(Tensors and nd-arrays are the same thing! So tensors are multidimensional arrays or nd-arrays for short.)
Indexes required | Computer science | Mathematics |
---|---|---|
n | nd-array | nd-tensor |
- A scalar is a $0$ dimensional tensor
- A vector is a $1$ dimensional tensor
- A matrix is a $2$ dimensional tensor
- A nd-array is an $n$ dimensional tensor
拓展
We often see this kind of thing where different areas of study use different words for the same concept.
索引
obvious: 访问一个多维数组, 需要几个索引
秩
即维数,或者说在张量中访问一个元素,需要的索引数
A tensor’s rank tells us how many indexes are needed to refer to a specific element within the tensor.
轴
An axis of a tensor is a specific dimension of a tensor.
tensor 属性
torch.dtype
Data type | dtype | CPU tensor | GPU tensor |
---|---|---|---|
32-bit floating point | torch.float32 | torch.FloatTensor | torch.cuda.FloatTensor |
64-bit floating point | torch.float64 | torch.DoubleTensor | torch.cuda.DoubleTensor |
16-bit floating point | torch.float16 | torch.HalfTensor | torch.cuda.HalfTensor |
8-bit integer (unsigned) | torch.uint8 | torch.ByteTensor | torch.cuda.ByteTensor |
8-bit integer (signed) | torch.int8 | torch.CharTensor | torch.cuda.CharTensor |
16-bit integer (signed) | torch.int16 | torch.ShortTensor | torch.cuda.ShortTensor |
32-bit integer (signed) | torch.int32 | torch.IntTensor | torch.cuda.IntTensor |
64-bit integer (signed) | torch.int64 | torch.LongTensor | torch.cuda.LongTensor |
torch.device
类型
- CPU
- GPU
指定GPU
1 | device = torch.device('cuda:0') |
注意
One thing to keep in mind about using multiple devices is that tensor operations between tensors must happen between tensors that ==exists on the same device==.
torch.layout
应该就是步长==?????==
总览
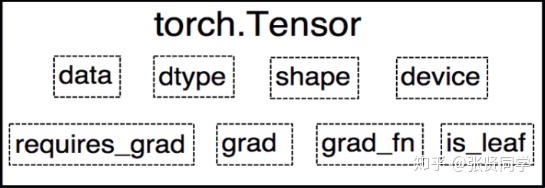
- data: 被包装的 Tensor。
- grad: data 的梯度。
- grad_fn: 创建 Tensor 所使用的 Function,是自动求导的关键,因为根据所记录的函数才能计算出导数。
- requires_grad: 指示是否需要梯度,并不是所有的张量都需要计算梯度。
- is_leaf: 指示是否叶子节点(张量),叶子节点的概念在计算图中会用到,后面详细介绍。
- dtype: 张量的数据类型,如 torch.FloatTensor,torch.cuda.FloatTensor。
- shape: 张量的形状。如 (64, 3, 224, 224)
- device: 张量所在设备 (CPU/GPU),GPU 是加速计算的关键
构建tensor的方法
直接构造tensor
torch.Tensor(data)
torch.tensor(data)
torch.as_tensor(data)
torch.from_numpy(data)
根据数值构造tensor
torch.eye(k)
: 生成 k 阶的单位矩阵torch.zeros(shape)
: 生成元素值都为 0, 形状为 shape 的张量torch.zeros_like(input, dtype=None, layout=None, device=None, requires_grad=False, memory_format=torch.preserve_format)
: 根据 input 形状创建全 0 张量torch.ones(shape)
: 生成元素值都为 1, 形状为 shape 的张量torch.rand(shape)
: 生成元素值随机, 形状为 shape 的张量torch.full()
1
torch.full(size, fill_value, out=None, dtype=None, layout=torch.strided, device=None, requires_grad=False)
- size: 张量的形状,如 (3,3)
- fill_value: 张量中每一个元素的值
torch.full_like()
1
torch.full_like(input, fill_value, dtype=None, layout=torch.strided, device=None, requires_grad=False, memory_format=torch.preserve_format) → Tensor
- input: the size of
input
will determine size of the output tensor. - fill_value: the number to fill the output tensor with.
- input: the size of
torch.arange()
: 创建等差的 1 维张量。注意区间为 $[start, end)$1
torch.arange(start=0, end, step=1, out=None, dtype=None, layout=torch.strided, device=None, requires_grad=False)
- start: 数列起始值
- end: 数列结束值,开区间,取不到结束值
- step: 数列公差,默认为 1
torch.linspace()
: 创建均分的 1 维张量。数值区间为 $[start, end]$1
torch.linspace(start, end, steps=100, out=None, dtype=None, layout=torch.strided, device=None, requires_grad=False)
- start: 数列起始值
- end: 数列结束值
- steps: 数列长度 (元素个数)
touch.logspace()
: 创建对数均分的 1 维张量, 数值区间为 $[start, end]$, 底为 base1
torch.logspace(start, end, steps=100, base=10.0, out=None, dtype=None, layout=torch.strided, device=None, requires_grad=False)
- start: 数列起始值
- end: 数列结束值
- steps: 数列长度 (元素个数)
- base: 对数函数的底,默认为 10
根据概率构造tensor
torch.normal(mean, std, *, generator=None, out=None)
: 生成正态分布 (高斯分布)- mean: 均值
- std: 标准差
4种模式
mean 为标量, std 为标量, 则==需要设置 size==
如:
torch.normal(0., 1., size=(4,))
mean 为标量,std 为张量
mean 为张量,std 为标量
mean 为张量,std 为张量
torch.randn()
和torch.randn_like()
: 生成标准正态分布。1
torch.randn(*size, out=None, dtype=None, layout=torch.strided, device=None, requires_grad=False)
- size: 张量的形状
torch.rand()
和torch.rand_like()
: 在区间 $[0, 1)$ 上生成均匀分布1
torch.rand(*size, out=None, dtype=None, layout=torch.strided, device=None, requires_grad=False)
torch.randint()
和torch.randint_like()
: 在区间 $[low, high)$ 上生成整数均匀分布1
randint(low=0, high, size, *, generator=None, out=None, dtype=None, layout=torch.strided, device=None, requires_grad=False)
- size: 张量的形状
torch.randperm()
:生成从 0 到 n-1 的随机排列。常用于生成索引。1
torch.randperm(n, out=None, dtype=torch.int64, layout=torch.strided, device=None, requires_grad=False)
- n: 张量的长度
torch.bernoulli()
1
torch.bernoulli(input, *, generator=None, out=None)
功能:以 input 为概率,生成伯努利分布 (0-1 分布,两点分布)
- input: 概率值
使用data的构造方法的不同
输出不同对比
code
1 | data = np.array([1, 2, 3], dtype=np.int32) |
output
1 | numpy: [1 2 3] ; dtype: int32 |
数据类型对比
torch.Tensor(data)
数据 | 数据类型 |
---|---|
tensor([1., 2., 3.]) | torch.float32 |
torch.tensor(data)
数据 | 数据类型 |
---|---|
tensor([1, 2, 3], dtype=torch.int32) | torch.int32 |
torch.as_tensor(data)
数据 | 数据类型 |
---|---|
tensor([1, 2, 3], dtype=torch.int32) | torch.int32 |
torch.from_numpy(data)
数据 | 数据类型 |
---|---|
tensor([1, 2, 3], dtype=torch.int32) | torch.int32 |
本质的不同
torch.Tensor() 和 torch.tensor()
torch.Tensor(data)
这是构造函数
torch.tensor(data)
这是工厂函数
You can think of the
torch.tensor()
function as a factory that builds tensors given some parameter inputs.相比于
torch.Tensor(data)
更好the factory function
torch.tensor()
has better documentation and more configuration options, so it gets the winning spot at the moment.
dtype 的对比
``torch.Tensor()`
使用的是默认类型
注意: 这个函数中的dtype也而==不可以==显式的设置, 如:
torch.Tensor(data, dtype=torch.int32)
❎other(
torch.tensor(), torch.as_tensor(), torch.from_numpy()
)使用的是推断类型(根据传入的原始数据, 自动推断出元素的类型)
注意: 这些函数中的dtype也而可以显式的设置, 如:
torch.tensor(data, dtype=torch.float32)
内存对比: copy vs share
代码
1 | data = np.array([1, 2, 3], dtype=np.int32) |
out:
1 | old: |
总结
Share Data | Copy Data |
---|---|
torch.as_tensor() | torch.tensor() |
torch.from_numpy() | torch.Tensor() |
- This sharing just means that the actual data in memory exists in a single place
- Sharing data is more efficient and uses less memory than copying data because the data is not written to two locations in memory.
torch.as_tensor() 和 torch.from_numpy() 的选择
- The
torch.from_numpy()
function only acceptsnumpy.ndarray
s - the
torch.as_tensor()
function accepts a wide variety ofarray-like objects
including other PyTorch tensors
张量操作
拼接
torch.cat()
将张量按照 dim 维度进行拼接
1 | torch.cat(tensors, dim=0, out=None) |
- tensors: 张量序列
- dim: 要拼接的维度
torch.stack()
将张量在新创建的 dim 维度上进行拼接
1 | torch.stack(tensors, dim=0, out=None) |
- tensors: 张量序列
- dim: 要拼接的维度
意义
使用stack
可以保留两个信息:[1. 序列] 和 [2. 张量矩阵] 信息,属于【==扩张==再拼接】的函数
例子
1 | # 假设是时间步T1 |
切分
torch.chunk()
1 | torch.chunk(input, chunks, dim=0) |
功能:将张量按照维度 dim 进行平均切分。若不能整除,则最后一份张量小于其他张量。
- input: 要切分的张量
- chunks: 要切分的份数
- dim: 要切分的维度
torch.split()
1 | torch.split(tensor, split_size_or_sections, dim=0) |
功能:将张量按照维度 dim 进行平均切分。可以指定每一个分量的切分长度。
- tensor: 要切分的张量
- split_size_or_sections:
- 为 int 时,表示每一份的长度,如果不能被整除,则最后一份张量小于其他张量;
- 为 list 时,按照 list 元素作为每一个分量的长度切分。如果 list 元素之和不等于切分维度 (dim) 的值,就会报错。
- dim: 要切分的维度
索引
torch.index_select()
1 | torch.index_select(input, dim, index, out=None) |
功能:在维度 dim 上,按照 index 索引取出数据拼接为张量返回。
- input: 要索引的张量
- dim: 要索引的维度
- index: 要索引数据的序号
torch.mask_select()
1 | torch.masked_select(input, mask, out=None) |
功能:按照 mask 中的 True 进行索引拼接得到一维张量返回。
- 要索引的张量
- mask: 与 input 同形状的布尔类型张量
t.le() t.ge()
1 | t.le(5) |
变换
torch.reshape()
1 | torch.reshape(input, shape) |
功能:变换张量的形状。当张量在内存中是连续时,返回的张量和原来的张量共享数据内存,改变一个变量时,另一个变量也会被改变。
- input: 要变换的张量
- shape: 新张量的形状
-1
表示这个维度是根据其他维度计算得出的
torch.transpose()
1 | torch.transpose(input, dim0, dim1) |
功能:交换张量的两个维度。常用于图像的变换,比如把 $chw$ 变换为 $hwc$。
- input: 要交换的变量
- dim0: 要交换的第一个维度
- dim1: 要交换的第二个维度
torch.t()
功能:2 维张量转置,对于 2 维矩阵而言,等价于torch.transpose(input, 0, 1)
。
torch.squeeze()
1 | torch.squeeze(input, dim=None, out=None) |
功能:压缩长度为 1 的维度。
- dim: 若为 None,则移除所有长度为 1 的维度;若指定维度,则==当且仅当该维度长度为 1 时可以移除==。
torch.unsqueeze()
1 | torch.unsqueeze(input, dim) |
功能:根据 dim 扩展维度,长度为 1。
数学运算
torch.add()
1 | torch.add(input, other, out=None) |
功能:逐元素计算 input + alpha * other。因为在深度学习中经常用到先乘后加的操作。
- input: 第一个张量
- alpha: 乘项因子
- other: 第二个张量
torch.addcdiv()
1 | torch.addcdiv(input, tensor1, tensor2, *, value=1, out=None) |
计算公式: $out_i = input_i + value \times \frac{tensor1_i}{tensor2_i}$
torch.addcmul()
1 | torch.addcmul(input, tensor1, tensor2, *, value=1, out=None) |
计算公式: $out_i = input_i + value \times tensor1_i \times tensor2_i$
bridge with Numpy
Tensor to numpy array
1 | tensor.numpy() |
函数
获取torch默认类型函数
1 | torch.get_default_dtype() |
suffix “_”
在函数的后面加上后缀 _
, 将会改变作用的数据
示例
1 | # source tensor |
out
1 | tensor([[1., 0., 0., 0., 0.], |
自动求导(autograd)
只要搭建好前向计算图,利用 torch.autograd
自动求导得到所有张量的梯度
torch.autograd.backward()
1 | torch.autograd.backward(tensors, grad_tensors=None, retain_graph=None, create_graph=False, grad_variables=None) |
功能
- 自动求取梯度
参数
tensors
: 用于求导的张量,如 lossretain_graph
: 保存计算图。PyTorch 采用动态图机制,默认每次反向传播之后都会释放计算图。这里设置为 True 可以不释放计算图。y.backward()
方法调用的是torch.autograd.backward(self, gradient, retain_graph, create_graph)
。但是在第二次执行y.backward()
时会出错。因为 PyTorch 默认是每次求取梯度之后不保存计算图的,因此第二次求导梯度时,计算图已经不存在了。在第一次求梯度时使用y.backward(retain_graph=True)
即可。create_graph
: 创建导数计算图,用于高阶求导grad_tensors
: 多梯度权重。当有多个 loss 混合需要计算梯度时,设置每个 loss 的权重。
retain_grad 参数
反向传播结束之后仍然需要保留非叶子节点的梯度
grad_tensors 参数
给 loss 设置权重
torch.autograd.grad()
1 | torch.autograd.grad(outputs, inputs, grad_outputs=None, retain_graph=None, create_graph=False, only_inputs=True, allow_unused=False) |
功能
求取梯度
参数
- outputs: 用于求导的张量,如 loss
- inputs: 需要梯度的张量
- create_graph: 创建导数计算图,用于高阶求导
- retain_graph:保存计算图
- grad_outputs: 多梯度权重计算
返回值
返回结果是一个 tunple
,需要取出第 0 个元素才是真正的梯度。
注意点
在每次反向传播求导时,计算的梯度不会自动清零。如果进行多次迭代计算梯度而没有清零,那么梯度会在前一次的基础上叠加。
故使用
w.grad.zero()
将梯度清零依赖与叶子节点的节点,
requires_grad
属性默认为True
叶子节点不可以执行
inplace
操作1
2
3
4
5
6
7## inplace 操作: 改变后的值和原来的值内存地址是同一个
a += x
a.add_(x)
## 非inplace 操作: 改变后的值和原来的值内存地址不是同一个
a = a + x
a.add(x)举例
1
2
3
4
5
6
7
8
9
10
11
12
13print("非 inplace 操作")
a = torch.ones((1, ))
print(id(a), a)
# 非 inplace 操作,内存地址不一样
a = a + torch.ones((1, ))
print(id(a), a)
print("inplace 操作")
a = torch.ones((1, ))
print(id(a), a)
# inplace 操作,内存地址一样
a += torch.ones((1, ))
print(id(a), a)结果自己跑一下嘛
问题
如果在反向传播之前
inplace
改变了叶子的值, 再执行backward()
会报错
逻辑回归
概念
二分类模型
模型表达式 $y=f(z)=\frac{1}{1+e^{-z}}$,其中 $z=WX+b$。$f(z)$ 称为 sigmoid 函数,也被称为 Logistic 函数
分类原则
逻辑回归是在线性回归的基础上加入了一个 sigmoid 函数,这是为了更好地描述置信度,把输入映射到 (0,1) 区间中,符合概率取值。
训练步骤
导入模型
计算误差 loss
后向传播 (call
loss.backward()
)加载优化器 optimizer: 注册模型中的所有参数
梯度下降 (call
optim.step()
)各参数的梯度保存在
.grad
属性中
反向传播
To backpropagate the error all we have to do is to loss.backward()
. You need to clear the existing gradients though, ==else gradients will be accumulated to existing gradients.==
模型构建总结
步骤
- 加载数据
- 定义神经网络
- 定义损失函数
- 训练网络
- 测试网络
PyTorch 构建模型需要 5 大步骤:
- 数据:包括数据读取,数据清洗,进行数据划分和数据预处理,比如读取图片如何预处理及数据增强。
- 模型:包括构建模型模块,组织复杂网络,初始化网络参数,定义网络层。
- 损失函数:包括创建损失函数,设置损失函数超参数,根据不同任务选择合适的损失函数。
- 优化器:包括根据梯度使用某种优化器更新参数,管理模型参数,管理多个参数组实现不同学习率,调整学习率。
- 迭代训练:组织上面 4 个模块进行反复训练。包括观察训练效果,绘制 Loss/ Accuracy 曲线,用 TensorBoard 进行可视化分析。
数据
数据模块
细分
- 数据收集: 样本和标签
- 数据划分: 训练集, 验证集和测试集
- 数据读取:对应于PyTorch 的 DataLoader。其中 DataLoader 包括 Sampler 和 DataSet。Sampler 的功能是生成索引, DataSet 是根据生成的索引读取样本以及标签。
- 数据预处理:对应于 PyTorch 的 transforms
考虑
- Who created the dataset?
- How was the dataset created?
- What transformations were used?
- What intent does the dataset have?
- Possible unintentional consequences?
- Is the dataset biased?
- Are there ethical issues with the dataset?
DataLoader
torch.utils.data.DataLoader()
1 | torch.utils.data.DataLoader(dataset, batch_size=1, shuffle=False, sampler=None, batch_sampler=None, num_workers=0, collate_fn=None, pin_memory=False, drop_last=False, timeout=0, worker_init_fn=None, multiprocessing_context=None) |
功能
构建可迭代的装载器
参数
- dataset: Dataset 类,决定数据从哪里读取以及如何读取
- batchsize: 批大小
- num_works: 是否多进程读取数据
- sheuffle: 每个 epoch 是否乱序
- drop_last: 当样本数不能被 batchsize 整除时,是否舍弃最后一批数据
Epoch, I’t’eration, Batchsize
- Epoch: ==所有训练样本==都已经输入到模型中,称为一个 Epoch
- Iteration: ==一批样本==输入到模型中,称为一个 Iteration
- Batchsize: ==批大小==,决定一个 iteration 有多少样本,也决定了一个 Epoch 有多少个 Iteration
举例: 假设样本总数有 80,设置 Batchsize 为 8,则共有 $80 \div 8=10$ 个 Iteration。这里 $1 Epoch = 10 Iteration$。
torch.utils.data.Dataset
功能
Dataset
是抽象类,所有自定义的 Dataset
都需要继承该类,并且重写__getitem()__
方法和__len__()
方法
__getitem()__
方法的作用是接收一个索引,返回索引对应的样本和标签,这是我们自己需要实现的逻辑__len__()
方法是返回所有样本的数量
数据读取
- 读取哪些数据:每个 Iteration 读取一个 Batchsize 大小的数据,每个 Iteration 应该读取哪些数据。
- 从哪里读取数据:如何找到硬盘中的数据,应该在哪里设置文件路径参数
- 如何读取数据:不同的文件需要使用不同的读取方法和库。
步骤
- 划分数据集为: 训练集, 验证集和测试集, 比例为 $8 : 1 : 1$, 并构造路径
- 实现
get_img_info()
和__getitem__(self, index)
,__len__()
函数 - 构建模型
DataSet
MNIST 数据集
损失函数
均方误差 MSE
$MSE = \frac{1}{m}\sum_{i = 1}^{m}{(y_i-\hat{y_i})^2}$
- $y_i$ 是预测值
- $\hat{y_i}$ 是真实值